Statistical Process Control (SPC) is a methodology used in industry to measure and control quality during the manufacturing process. Quality data in the form of product or process measurements is obtained in real-time during manufacturing. This data is plotted on a graph with predetermined control limits. Control limits are determined by the capability of the process, while specification limits are determined by customer needs.
Statistical Process Control
That is, in its most basic form, SPC is a systematic approach to collecting and analyzing process data for prediction and improvement purposes. SPC is about understanding process behavior so you can continually improve results.
As you learn about SPC, you will come across terms that describe central tendency:
- Mean (the arithmetic average)
- Median (the middle point)
- Mode (the most frequent)
On the other hand, you will also find terms that describe the width or spread of the data:
- Variation: A term used to describe the amount of spread in a data set.
- Range: A measure of spread that is equal to the maximum value minus the minimum value of a given data set.
- Standard deviation: a measure used to quantify the spread of a data set from its mean value
Origins of Statistical Process Control:
Shewhart Statistical Process Control (SPC) Charts
Dr. Walter A. Shewhart (1891–1967), a Bell Labs physicist who specialized in the use of statistical methods to analyze the random behavior of small particles, was responsible for the application of statistical methods to process control. Until Shewhart, quality control methods focused on inspection of finished products and classification of nonconforming products.
As an alternative to inspection, Shewhart introduced the concept of continuous inspection during production and plotted the results on a time-ordered graph that we now know as a control chart. By studying the patterns of plot points, Shewhart realized that some levels of variation are normal, while others are anomalies.
Using known knowledge of the normal distribution, Shewhart established limits to be drawn on graphs that would separate expected levels of variation from the unexpected. He later coined the terms common cause and assignable cause variation.
Dr. Shewhart concluded that every process exhibits variation: controlled variation (common cause) or uncontrolled variation (assignable cause). He defined a process as controlled when "through the use of experience, we can predict, at least within limits, how the process can be expected to vary in the future."
He went on to develop descriptive statistics to aid manufacturing, including the Statistical Process Control Chart, now known as the X-Bar Chart and Range (Xbar-R). The purpose of the Shewhart Statistical Process Control Chart is to present distributions of data over time to allow processes to improve during production. This chart changes the focus of quality control from defect detection after production to defect prevention during production.
Why use Statistical Process Control in manufacturing?
Today's consumers expect the best quality products at the lowest price. Why do manufacturers use SPC? Because Statistical Process Control can help you meet both demands.
By using statistical process control, you as a manufacturer can move from a detection approach to a prevention approach, reducing or eliminating the need to rely on grading or inspection. Statistical process control on your assembly line can increase productivity, reduce waste, and reduce the risk of shipping subpar products.
Statistical process control reduces scrap
For many years, the term quality control meant inspecting to eliminate unsatisfactory products. Products are produced and then inspected to determine whether or not they are fit to be shipped to the customer. Those that are not acceptable are discarded or reworked. Product grading is not only expensive (since you're paying one employee to make the product and another to make sure the product is correct), it's also not very accurate. Studies have shown that 100% inspection is approximately 80% effective.
The SPC helps manufacturers break out of this inefficient cycle. It leads to a system of preventing suboptimal products during the production process rather than waiting until products are complete to determine if they are acceptable. This reduces waste, increases productivity, makes the product quality more consistent, and reduces the risk of shipping nonconforming products.
When SPC is properly implemented, manufacturers foster an environment where operators are empowered to make decisions about processes. In this way, processes and product quality can be continuously improved.
SPC is a powerful tool, but success depends on regular and proper application. Management must support its implementation through employee trust and education and a commitment to supply the necessary resources.
Implementation of Statistical Process Control
Statistical process control is certainly not the only technique used to improve processes. But for our purposes here, we will focus on two of the most used tools in SPC:
- Histograms
- Control charts
Histograms
Histograms can provide a quick view of process variation and are used to plot frequency distributions.
Control charts
Control charts are the most popular tools associated with SPC.
Control charts are used to determine whether a process is stable or unstable. There are many types of control charts that can be used to accommodate the nature of different types of data streams and sampling methods.
While SPC charts are revealing, manufacturers today are increasingly recognizing the benefits of moving away from manual SPC, done by recording data on paper and then running analyzes through offline spreadsheets or statistical software, and instead of using SPC software.
Would Statistical Process Control software be useful on the assembly line?
A quality control software for manufacturing can offer you multiple benefits if you install it on the assembly line:
- Show relevant information faster.
- Filter data based on role (eg operator, quality manager) and location (eg lines worked that day).
- Faster, more focused, and more detailed analysis.
- Alerts and targeted notifications.
- Enterprise-wide mobile visibility of operations.
Remember that using Statistical Process Control just to find an out-of-control point on a control chart and then determining and eliminating the assignable cause is not the same as developing continuous improvement. SPC can be fully realized only when you use it to improve processes and reduce variation.
Types of process variation
There are two types of process variation:
- Common cause variation is inherent in the system. This variation can only be changed by upgrading equipment or changing work procedures; The operator has little influence over it.
- Assignable cause variation comes from sources outside the system. This variation can occur due to operator error, use of improper tools, equipment malfunction, raw material issues, or any other abnormal disruptive input.
The goal of statistical process control is to understand the difference between these two types of variation and to react only to assignable cause variation.
When a process is target-focused and in a state of statistical control, any adjustments to the process only increase the variation. Adjusting a process that is in control is known as manipulation.
The funnel experiment and Deming's four rules
The classic analysis of the effects of manipulation is the Deming Funnel Experiment. In this experiment, participants throw marbles through a funnel suspended above a target. The funnel represents the process, the location of the marble droplet is the feature being produced, and the target is the customer's specification.
Deming described four approaches, also known as rules, that encompass the typical ways in which the funnel is manipulated by experiment participants (Out of Crisis, 1986, p. 328).
Rule 1: no adjustment
The ideal approach is to leave the funnel fixed and aimed at the target, without making any adjustments. When a process is stable, focused, and shows only inherent variation, there is no reason to adjust.
Conclusion: Before attempting any tuning of the process, you should gather enough data to make sure you understand the normal behavior of the process. Use a control chart to track variances and then adjust the process only when special variances occur.
Rule 2: Adjust from the last position
Sometimes known as the "human nature" approach, some participants move the funnel after each drop, trying to compensate for variation from the previous drop. In this approach, the funnel moves the exact negative distance from the drop. Compensating for the "error" of the drop might improve the average on target, but double the variation.
Conclusion: When the participants compensate for the error, the variation doubles, and remember, variation is the real problem. This problem is prevalent in meter calibration when manufacturers adjust a meter after taking a standard measurement.
Rule 3: Adjust from the target
Participants trying to take a "logical" approach also move the funnel to try to make up for the earlier drop. But in this case, the funnel doesn't move based on its last location, but rather its distance from the target. For example, if the previous drop measurement was 5 units above the target, participants move the funnel 5 units below the target.
Conclusion: Although this approach seems logical, it results in an oscillating process.
Rule 4: Adjust from the last drop
In this approach, participants move the funnel to point to the previous drop rather than the target. In other words, on drop “n”, they set the funnel over the location of drop n-1. As you might expect, this approach creates a pattern that is constantly moving away from the target.
Conclusion: Believe it or not, this approach occurs in calibration scenarios when a product is used to configure the next production. This problem is typical in workplaces where on-the-job training is prevalent.
Location: Measure of central tendency
As we told you at the beginning of the article, there are three measures of the central location or trend of the histogram:
- Mean: the arithmetic average of a set of collected values.
- Mode: the value that occurs most frequently within a set of collected values.
- Median: the value that defines where half of a set of collected values is above the value and half is below.
When compared, these measures show how the data clusters around a center, thus describing the central tendency of the data. When a distribution is exactly symmetric, the mean, mode, and median are equal.

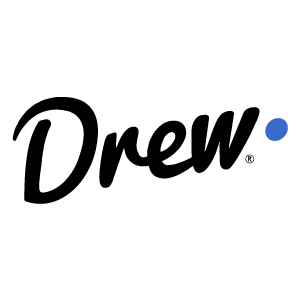
¿Nos dejas un comentario?